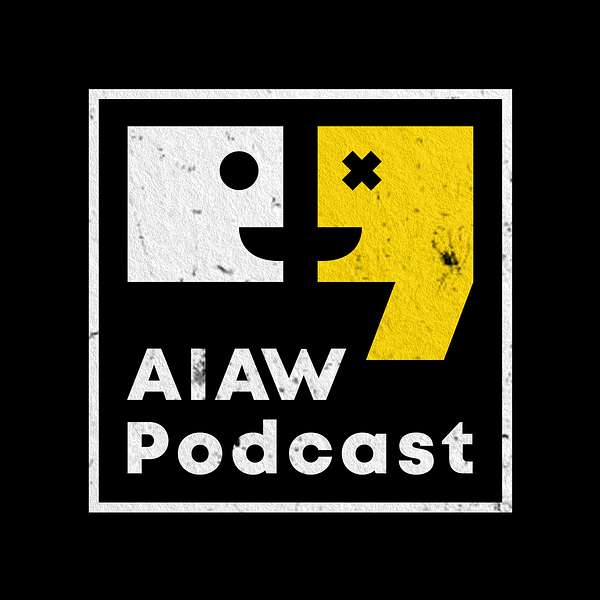
AIAW Podcast
AIAW Podcast
E139 - Revolutionizing Healthcare with AI - Anders Eklund
Unlock the future of medical AI with insights that could reshape the way we approach healthcare. We're joined by the brilliant Anders Eklund from Linköping University to explore how AI is revolutionizing radiotherapy for brain tumors. Discover how recent advancements in AI are slashing the time for tumor segmentation and treatment planning from hours to minutes, all while enhancing patient safety. Through innovations like MRI-based synthetic CT scans, we're witnessing a significant reduction in radiation exposure, offering a safer path forward in medical treatments.
We further journey into the fascinating world of federated learning and its critical role in maintaining data privacy in medical imaging. Anders Eklund shares his expertise on the transition from traditional methods to cutting-edge machine learning, emphasizing the importance of merging domain expertise with technological advancements. Find out how federated learning is paving the way for secure data sharing, allowing model updates without the need for centralizing sensitive information, and why this approach is a game-changer for healthcare data privacy.
Our conversation takes on the complexities of regulatory landscapes and the future of AI in society. We ponder the balance between innovation and compliance, questioning whether stringent regulations could stifle groundbreaking developments in AI applications. From the potential of AI to perform complex tasks autonomously to its role in interdisciplinary collaborations, we explore the far-reaching implications of these advancements. Join us as we not only highlight the promising future of AI across fields but also confront the challenges and ethical considerations that accompany this technological revolution.
Follow us on youtube: https://www.youtube.com/@aiawpodcast
Both the.
Speaker 2:ASIST and then you had another call that you also want to apply for. Yeah, can you just elaborate a bit more? What was the ASIST project?
Speaker 3:So the ASIST project was about mainly image-guided therapy. So radiotherapy is one type of image-guided therapy where you use medical images to decide how to use radiation to kill cancer cells. So the ASIST project has several different parts, but we mainly focused on brain tumors in relation to radiotherapy.
Speaker 2:And then using AI to detect tumors in different ways, or what was AI used for primarily?
Speaker 3:Yeah, so in radiotherapy there are several steps that take a lot of time for the doctors. So they have to draw the tumor, they have to draw different risk organs, so that can take several hours for one patient and then from that they have to generate a treatment plan right, which can also take hours. But with ai and deep models you can reduce that to maybe five, ten minutes yeah, this is something an augmentation boost that is very, very strong so yeah, empowerment empowerment augmentation.
Speaker 1:I mean like the person augmentation.
Speaker 2:you think sometimes in data or AI augmentation, so I think that term can be a bit confusing.
Speaker 1:Yeah, okay.
Speaker 2:Fair enough.
Speaker 1:But there is a workflow here that has some key points that are very substantial that we can support here.
Speaker 3:Yeah, there is a clinical workflow with what we call segmentation and treatment planning, and it can take several hours per patient so you guess you use semantic segmentation techniques.
Speaker 3:Then yeah to do formally the kind of encirclement of the tumor or the area where the potential tumor exists yeah, so that the tumor that you want to kill with radiation and then the risk organs that you don't want to damage, so that becomes an interesting optimization problem. So how do we kill the tumor while not damaging other parts of the brain or whatever organ you're looking at?
Speaker 2:And you also used AI for the treatment plan generation or did you focus on yeah?
Speaker 3:so you can do that with AI as well. So you can go from the segmentations to predict how gamma knife or a LINAC that will apply the radiation.
Speaker 1:So, if I understand it right here, that is not a statement of you guys. It's like first we do AI in order to optimize the optimization problem on the segmentation of cutting out exactly right, and then you can understand and predict how much gamma ray should we shoot, and then you need to have a treatment plan.
Speaker 3:you know how will you space out that shooting the gamma rays, so to speak. Yeah, so you can use ai in to. I mean, if we take it from the beginning, in radiotherapy, the traditional way is that you collect CT images, or basically 3D x-ray, but that requires radiation which is bad for the patient. So now we are moving more and more to MRI, which does not require any radiation, but you sort of still need the CT to calculate the best radiation plan, because CT is sensitive for bone and you can use it to calculate how the radiation will be reduced when it hits your bone, for example. Interesting, so you can also generate instead of collecting the CT, you can generate synthetic CT from the MR images.
Speaker 1:So that's another step where you can use AI and all of a sudden now, things that take hours to do manually, we are now finding ways how we do it algorithmically, but not just time-wise then, it's also a health benefit, right?
Speaker 2:So you can use an MRI which is less damaging to the patient. And then from that generate CT scans which you then can use to have the proper treatment plan for that.
Speaker 3:Yeah, so then you remove the radiation for the patient Interesting. But there was another angle here.
Speaker 1:And what we talked about is just before we started the pod. You're moving actually in a direction now where there have been steps or projects that leads into assist, and one of the key learnings that you sort of are addressing in the assist project was how do we create bigger data sets in a good way? So there is a piece here that we will talk about today. A lot federated learning, yeah. So what was that piece in in the assist project about collecting the data sets? Could you frame that?
Speaker 3:well, it's a common problem for basically all types of medical data that if you want to do deep learning in ai you have you usually have to have big data sets, but in medicine that's usually difficult because you have to respect ethical rules, gdr and other regulations.
Speaker 1:You can't just take all the data and then you stump it.
Speaker 3:No, you cannot just call a hospital and get 10,000 images, which is probably good Cool.
Speaker 2:And I think this is a topic we will dig much deeper into very soon. But just to also talk a bit about the other point, you had another deadline today to submit some other application.
Speaker 3:Yeah.
Speaker 2:Can you just elaborate quickly on that? What was that application that you applied for?
Speaker 3:Yeah, that's basically to continue the federated learning part of the ASSIST project. So then we wrote an application to a call from something called WASP DDLS. So WASP is a program from Wallenberg Foundation and DDLS is another program from the same foundation, focusing on life science. So they wanted applications where you where between three to five researchers go together to create a nest that will achieve something bigger together. So we wrote an application with five persons from five Swedish universities in five different cities so that we can continue this federation in five cities.
Speaker 1:And then I assume Andreas Hellander, or who was the guy, or Salman Tor, or who are you working on? That has a scale out connection.
Speaker 3:Yeah, Andreas and Mattias Åkesson.
Speaker 2:Well, I wish you the very best luck on that application. It's a super important field and you have so many interesting parties and companies and Linköping on the radar as well. So congrats on that, and I hope you can relax a bit now the day is over, and now you're here to speak on the podcast and just have a bit of fun.
Speaker 1:Yeah, but how did you manage? Did you have some friends?
Speaker 3:Because you were up here in Stockholm and I guess people were working to get their report in and report out yeah, I mean, I, I tried to be, I tried to plan a lot ahead, yeah, which helps if you have to write these big applications. So for, for this application we submitted today, we needed or we ended up with, 19 support letters, so then you have to start at least a month ahead to ask for them because people are busy. I was going to say chat GPT. Yeah, how much chat GPT was used in the application.
Speaker 3:You cannot sign the letters, unfortunately.
Speaker 2:Well, I hope you got some useful chat GPT as well in the writing process for the application.
Speaker 1:But a big day, an exciting day, yeah.
Speaker 2:Well, anyway, you know, super welcome here Anders Eklund, your associate professor right at Linköping University, the best university in the world, I can say, coming from that place myself a bit biased, but still I think from an AI point of view, the best university. A lot of people would disagree with me.
Speaker 1:You could have so much shit for that comment, by the way.
Speaker 2:This is objective feeling, but it's a really good university, I think. Anyway, you focus on a lot of different things, but we're here to speak a bit more on federated learning and how we can work with data privacy in machine learning field in different ways, so that will be super interesting to dig more into but before that can we just but just one second the theme we said even in, more interestingly, federated learning, specifically in the health or medical sector, because it opens up a lot of different challenges where federated machine learning and privacy comes in.
Speaker 1:I just wanted to add the medical part here In medicine there's lots of sensitive data.
Speaker 2:Yeah, so I like that, I think it's nice to be really concrete, as you say as well, to have a concrete field like medical field to speak about. It's very obvious why we need this kind of privacy-preserving data management in different ways.
Speaker 1:But before that.
Speaker 2:Perhaps Anders can you just elaborate a bit more. Who is really Anders Eklund?
Speaker 3:How would you describe yourself Well, a person who likes to do research. So I mainly develop methods for analyzing medical images. So, for example, my main background is in image processing and then I've also done statistics for image data. And then the image processing field has become more and more machine learning, so now it's like 90 or 95% machine learning.
Speaker 2:Were you a bit. I mean, I know some other field or people that were in academic research and they spent so much time doing like edge detection algorithms back and forth, non-deep learning based, and then they got completely side rails by deep learning and all the research had gone to waste in some way. Was that something that happened to you as well?
Speaker 3:A bit like that. But I mean I try to see the positive side that now I can learn about. I have a reason to learn about machine learning and I think that many times you can take inspiration from old methods to make machine learning smarter, and not just through in-data.
Speaker 1:Oh, this is a topic. You know combining, you know symbolic and machine learning, or understanding how we take the next step.
Speaker 2:We have so many different angles, don't say symbolic, I would say, but domain expertise, at least Domain expertise pins.
Speaker 1:We have the idea of physical informed neural networks. We have many ideas like that. Don't throw away the old.
Speaker 3:Yeah, I think. I mean I have one course in medical image analysis that starts in November every year and I mean, from one perspective I should transform that into a pure machine learning course. But from one perspective I don't want to do that.
Speaker 1:Okay, interesting.
Speaker 3:Because I think it's important to first learn the older methods. Right, okay, cool, because there are tons of online courses on machine learning and but learning more traditional image processing it's maybe not as easy but and why?
Speaker 1:why would that be? A benefit to understand that angle. What you're up, you know what, why is? Why is it relevant?
Speaker 3:well, I mean, it depends on the problem you're trying to solve. But sometimes you don't need machine learning. You can just use simpler methods or a combination of simpler and more advanced methods so should I understand this a little bit?
Speaker 1:like these fundamentals, you need to get around image processing, even to be mastering how to apply machine learning yeah and and maybe to.
Speaker 3:I mean, instead of just starting with machine learning, start with some simple method and see how well, does that?
Speaker 2:I think it's an important topic. I got a question actually today from a person that were working with a project and they come and asked Anders, is it okay if I don't use machine learning for this one? I mean, I know that that was the the idea, but but I think we can use you know, simpler statistical methods, and is it okay if we do that? That's great. Don't don't apologize if we can find a similar way to to solve the.
Speaker 1:that's great.
Speaker 2:Don't apologize for that If we can find a similar way to solve the problem, that's even better, right?
Speaker 1:Well, we have Einstein's razor, Occam's razor, you know.
Speaker 2:It's strange how so many people just you know always use the sledgehammer, so to speak, for things that may not be needed sometimes, and you should be really happy if you can find a way that can use simpler methods to solve the problem.
Speaker 1:Wouldn't you agree, yeah, yeah. But then Anders is onto something, because if we only teach the sledgehammer, you can use generative AI for everything you know, llm for everything. Oh, that's going to be costly, yeah, I mean especially generative AI.
Speaker 2:Everyone is just saying how is an LLM going to solve this? This has nothing to do with LLM.
Speaker 1:You could, but that's going a big, big roundabout. We are on your side. We think you should continue doing that.
Speaker 3:I mean we have some parts that are deep learning, of course, but another aspect that this is sort of the last course that the students take before doing their master thesis, and in their master thesis many do deep learning, so then they will learn that part anyway. So then it's maybe better to focus on more traditional methods.
Speaker 2:How did you get started into academia? How long ago? What was your initial kind of steps into? I?
Speaker 3:started my PhD in 2008. So I young person. I first worked like half a year as a consultant at the company, but it felt like I couldn't use everything or much of what I learned as a student. So then I applied for a PhD position and I didn't know much about it, but I applied and then I got stuck.
Speaker 2:What was the title in the end for the PhD thesis that you had?
Speaker 3:What was the title in the end for the PhD thesis that you had? I think it was computational medical image analysis with an application to what? Was it real-time fMRI, non-parametric statistics or something Interesting?
Speaker 2:Great. And if you were to just elaborate a bit more what's your current position at the university, what's your role and what do you work with mainly now?
Speaker 3:Well, right now I try to find more money to hire new people.
Speaker 1:So honest, so honest. I love it.
Speaker 3:No, but I have a mix of teaching and research and I'm also the director of graduate studies at our department and I work at two departments. So the main department is the Department of Biomedical Engineering, which is located at the hospital, and then I also work at the Department of of computer science, where it's more focused on statistics and machine learning awesome is one more applied and more, one more methods, when, if you contrast where your work is going or what your focus is going yeah, I, I mean at At Hospital.
Speaker 3:I collaborate with several medical doctors and it's almost another language when you talk to them. You cannot really talk about gradient descent and atom optimization, but you have to talk more about the problem they're trying to solve. But at the other department it's more on the theory and making new methods.
Speaker 1:I have a joke for you. Imagine working on the same project and one guy is speaking Latin and another guy is speaking Python. That's what you're doing.
Speaker 3:Yeah, I mean, if you want to work with medical doctors as an engineer, you have to be prepared that it will take some time, maybe several years, before you really understand each other.
Speaker 2:Yeah, because it's he has some medical parents I have medical parents.
Speaker 1:I grew up with latin as my, you know, breakfast table. Yeah, but I think there, I think there's a profound truth in what you're talking about. When people are trying to build projects and we are trying to, you know, in enterprise, typically IT tech roles is over here, business is over here, and then you're supposed to solve this ping pong and you're now saying and I've used this analogy, this joke, you know, of course you will have if you want to work on cancer spotting an image, old example you know, of course you have the research team with the Python-speaking guy together with the Latin-speaking guy in the same team. You know, don't even try this at home, kids, if you're not working together. And I think you said it right now a couple of years for this lingo to emerge. So what happens if I go to enterprise and we are ping-ponging in projects and we are never really working together? It's futile, it will never work. Do you agree? I mean, like this is a hardcore problem.
Speaker 3:Yeah, I think you have to work close together for.
Speaker 1:Period.
Speaker 3:Yeah, continuous. But I think that the cool part is that we can learn from each other. Yes, so that they learn more and more engineering and I learn more and more medicine. So I think it's interesting that you can learn something not by taking a course, but just working with someone who really knows that topic.
Speaker 2:Yes, that's much more so your skills as a machine learning expert grows by working in medicine, and medicine expertise grows by working in machine learning?
Speaker 1:yeah, in one way, but I this is so big topic because I think the misunderstanding here is how this collaboration pattern works that we can ping pong and if you use the word now, this is co-creation. So we are not ping ponging between two disciplines, we are merging them and we are iterating together to create a new normal. So the new normal is in the middle of the Venn diagram of two different domains. It's intersectional innovation and this, to me, is co-creation.
Speaker 3:So you need to learn them, they need to learn you, and the ping-ponging with two separate domains will not get the same result and I think a lot of mistakes are done in this area if I go to enterprise, as an example, yeah, yeah, but that's one reason why it's so important that our department is located at the hospital Exactly, so we can easily go to them or they can come to us.
Speaker 1:It's the right move for sure, awesome.
Speaker 2:Should we move a bit more into the core topics in the evening and working in the medical field? Of course, we have a lot of sensitive data and we still want to apply machine learning techniques in different ways, and that entails that we need to have some kind of secure way of managing that data, and thus we enter into the field of federated learning. Perhaps you can start a discussion. How would you describe what federated learning is?
Speaker 3:basically a way to, for example, train models with more data without having all the data in one place.
Speaker 2:So the traditional approach is you basically collect all the data, put it in one place, put it in the training data set and you can train the model from it. But now you don't need to merge all the data.
Speaker 3:Yeah, you can have data in different hospitals or different cities, which makes it you don't have to send the data anywhere. That's the main advantage.
Speaker 2:So could you phrase that, instead of sending data to one place, you send the models to where the data is?
Speaker 3:Yeah, like if you want to find the best recipe of a certain cookie or a cake and maybe you have bakers in three or four cities, you know that the milk in one city is different from the milk in another city and the eggs are a bit different and you don't want to send around the milk and the eggs between the cities, but you can send around the recipe, which is much easier.
Speaker 2:So in practice, and I guess federated learning can be used for different use cases. One is for large hospitals, for example, as you were speaking about, but it could also be like small edge devices like mobile phones or something that could be using it, like at the Google keyboard, for example, that can be learning how to predict the next word without having to send the data from each mobile phone into some kind of central place.
Speaker 3:Yeah, and you can maybe have it in every car for some, if there are applications where you need data from every car.
Speaker 2:So, in short, move the model instead of moving the data.
Speaker 1:Yeah, and this is I think this is a massive discussion. I grew up in the energy industry so I first time I came across and met Andreas at the University of Uppsala, I realized how big this is going to be in the energy industry, that we have our different distributing service operators and we have a transmission service operator At one end side.
Speaker 1:I was supposed to not share any data because it was NSI class this is critical infrastructure in Vattenfall but at the same time, I need to send some data in order to optimize the grids of the whole thing. So here we have now a classical problem that we have many different competitors in one hand that should have their data and do their thing, and at the same time, you need to share in order to optimize for the greater good. So there are so many examples like that.
Speaker 2:If you start thinking about it.
Speaker 1:It's a massive problem. Logically, why should different people in different companies send all their data to a central?
Speaker 3:There are so many angles in this. Yeah, I mean in medicine. You can think of medical images, you can think of genetics, you can think of text in patient records. So there's many examples where you have sensitive data.
Speaker 1:And the whole journal problem. We are trying to coordinate to have a perfect patient flow and everybody thinks why don't you fix it? And then, on the other hand, we have the ethical consideration of privacy, and how do we share data and all that, which is not an easy balancing act here. So to find techniques where you don't need to go beyond, you can have privacy-preserving approaches. It makes total sense.
Speaker 3:It makes total sense, yeah, but it's also you also have to follow the Swedish law. So I'm not an expert on legal aspects. But for research you can do basically whatever you want, as long as you have ethical approval. But as I understand it, there are sort of other laws if you want to do it in clinical practice. So if you manage to show in the research project that federated learning works, we can do many things. But I think that the Swedish law says something like that you cannot combine data from different hospital regions, so then it may not be so easy to use it in clinical practice and just to make it really simple and clear, I mean we have this kind of cross silo, cross device type of federated learning.
Speaker 2:ensure it when you do traditional machine learning, you you feed batches of data to a model, you calculate some kind of loss value, you get some kind of gradient that then you use to update the parameters of a large model in some way. Now, I guess, instead of calculating the batch and the loss in a centralized place, you calculate the loss outside, in the edge, in a university or some device, and then you update the model just locally at that place, right?
Speaker 3:Yeah, each node in the federation will do one or a few batches of training and then they will send an update to like a global server which will combine the updates from all nodes and then merge them somehow into a new model that will be sent out again. And then you iterate this, that you send the model back and forth all the time.
Speaker 2:And that has some pros and cons. I guess the pros, of course, is that you don't have to send the data and you have better privacy preserving methods in this case, but I guess there are some cons as well, right? What's the drawbacks of using federated learning? You would say.
Speaker 3:Well, it will be more. It can be more difficult if the data in every city or in every hospital is a bit different. If you have heterogeneous data that maybe have maybe the patients are older in one city compared to another, or maybe you use different scanners in every city to collect images Then the images will look different, so they have different statistics. So then there are ways that you can sort of harmonize the data. But then the challenge is to harmonize the data without sending any data around. So then you sort of go back to square one any data around. So then you sort of back to square one that you want to make the images or the data more similar, but without sending it around.
Speaker 1:For me that is not as technical as you guys, is there a way you can help me sort of break this bigger problem down into the core patterns or the core problems we need to solve? I mean like, okay, problems we need to solve. We want to have privacy preserving data. We want to send the model to the data? Great, get that part. Read the memo. How do you now break that down into a workable solution? What are the core patterns or capabilities that we are trying to solve here, underneath the surface, so to speak?
Speaker 3:To run a federation?
Speaker 1:Yeah, as an example.
Speaker 3:Well, you need like a computer in every city or every hospital, okay, and then you can have a challenge that the hospital may not want to open the firewall. Okay, so either you need to have the computer outside the firewall okay. So either you need to have the computer outside the firewall or you need someone to open the firewall for you so you have one problem here.
Speaker 1:One piece of the puzzle is to solve the compute at the site yeah, and then you.
Speaker 3:I mean, when we did federated trainings between cities, then I'm sure you can optimize this, but then we sort of needed all the persons to start their clients at the same time, and then the more nodes or cities you have, the more the harder it will be to synchronize that everyone can work at the same time.
Speaker 1:So there's a coordination synchronization topic here somewhere.
Speaker 3:Yeah, so there was a paper published one or two years ago where they did federated learning with 71 cities around the world, and then you start to have different time zones. So if you want to have different time zones, so if you want to call someone in Japan, they may be asleep or so. Then you have this logistics problem that you need everyone to start, basically their clients, at the same time is this as a way to get the data into the training?
Speaker 1:run in a good way. Why do you need to do it? Why can't you do it as a synchronous?
Speaker 3:well, I guess you can do it in different ways, but at least you have to make sure that all the computers are up and running. So in the best case you can control all the computers from one computer and then you don't have this problem. But it can be a challenge if you run into small hurdles, that you need to restart your computer or fix some small bug, then several persons need to do something at the same time persons need to do something at the same time.
Speaker 2:Okay, so some technical challenges and, I guess, security challenges there, because universities have really strong cybersecurity demands on them. It's not easy to get into their on-prem networks, it's not easy to send something out of their on-prem networks, but you do need to share not the data but the models or the gradients in some way, and that in itself could be challenging, I guess, and then the coordination around that. But if we were to move to a question, how safe is it to share the model or the gradients, in contrast to the data? Is there some kind of privacy or legal challenges with that?
Speaker 3:yeah, I. I would say that it depends on, uh, how much training data you have at each site and it depends on how big is the model or how many trainable parameters right, because the more parameters it has, the more likely is that it will just memorize your training data. So there are more papers coming out showing that you can, from the model, extract the training data. So that's one topic that we want to cover in this application we submitted. So can we quantify data leakage? Can we quantify how much has a model memorized from the data?
Speaker 2:And I guess model leakage in some ways. So can you extract the data from a model itself.
Speaker 3:Yeah.
Speaker 2:And if you can extract private or personally identifiable information, it also becomes a legal issue or challenge.
Speaker 3:Yeah, I think about one or two years ago there was an investigation by the Swedish EMI, or the Swedish Authority for Privacy Protection, where they looked into this. Oh yeah, Because I think there was a project between Gothenburg and Halland where they did federated learning with tabular data and then they wanted to investigate can we do this in a clinical practice? And then they wanted this. Emi investigated this and I think that they came to the conclusion that you can never be 100% sure that there's no data leakage. So then the machine learning model itself can be seen as personal information. So they had a rather strict view of this, which I think is too strict.
Speaker 1:Because if you have this super strict view, it will tie down anything in the end, right?
Speaker 2:And you can't do anything.
Speaker 1:You can't do anything, but maybe okay, can we go a little bit more understanding on the patterns, because we've talked now about the edge patterns of collecting data. What is happening on the other side? What is the difference? Figuring out how to build a model that is working monolithically versus distributed, or something?
Speaker 2:like that.
Speaker 1:What are the core problems here? Do we have a summary on that?
Speaker 3:Can you give an example?
Speaker 1:I was just elaborating that when you build a model and you're working with one training data set and it's all in here and everything is super easy because the data set is clear, now, okay, if I have a central model, I'm going to send the model out, I'm going to collect that back. There's some extra mechanisms here that you kind of don't need to do when you do a monolithic approach on this. So I just wanted to elaborate what are those mechanisms, what are those patterns that sort of make something work distributed on the hub side.
Speaker 3:Yeah, well, one thing is that you need to define how you should merge all the models. Good one, so the easiest is just to take the average. Like, if you have a model with one million weights, then for each weight just take the average over all models. But that's very simple, because if maybe you have one node with 1,000 data points and maybe one node with 25 data, points.
Speaker 3:So then it's very unbalanced. Exactly. So then you need to put weights on a higher weight on the big node and a lower weight on the smaller node.
Speaker 1:So what we're talking about here is like we're talking about different kinds of biases or different kinds of balancing. So when we're working distributed, now we have the thing is okay. We're going to put this together now and it should be as truthful as possible, yeah.
Speaker 3:Federated learning solves the big problem of data sharing, but it will create other problems that we need to solve. And then, as I mentioned, we have heterogeneous data, that they may look different, so that a model that you train in one city may work well for that city, but a local model train in another city will work well for for that city. So if you take the average, you may get something that doesn't work anywhere because your data are too different.
Speaker 1:Yeah, but this is exactly what I was trying to go for. So, when we go distributed, what are the key problems underneath here? So you highlighted now getting the data in from all the different places, the heterogeneity problem, the balancing problem? So, there are three or four fundamental topics, that sort of pops up. We're getting benefits over here, but federated machine learning means we are solving this stuff.
Speaker 3:Yeah, and if you have lots of data in one place and not so much data in another place, and let's assume that you have the same hardware in every city, it means that the training will take much longer, and it means that in the other city you train for maybe one minute and then you're just waiting for the other city to finish. So then it becomes more inefficient because that all the nodes have to wait for each other. So then you can. You can, for example, uh, randomly say that, okay, we skip the city for a few rounds, so we do more training here.
Speaker 1:Yes, yes, to reduce the waiting time yeah, so the interesting thing is like going distributed, going federated, has a no-brainer we. This is stuff we need to solve but, it's not something that is easy, but when you fix that, you now need to take care of this.
Speaker 3:You get other problems, but that's good for me as an engineer because then I have a job to do. Yeah, but I think also I'm not saying this is bad.
Speaker 1:It's just about what is the engineering that goes on here, and this is not I mean like. So we have like examples like ScaleOut right that builds technologies and platform. About this exactly because of these problems, because when you open up this can of worms, there are things that need to be solved and they are not solved in typical monolithic architecture from the vendors.
Speaker 2:You call yourself an engineer, by the way. Do you consider yourself mainly an engineer or a scientist?
Speaker 3:I guess a mix.
Speaker 2:But I mean, I suppose most engineers they like to solve problems, Do you have a preferred definition of what science and engineering is Any differences between them?
Speaker 3:I have some, but it will be fun to hear yours. Not from the top of my head, no.
Speaker 1:Well, we had this conversation a couple of times. What is the difference? It's a very nice angle actually.
Speaker 2:I can tell you mine and see what you think about it, and I think there is a lot of overlap between the two, but there is a clear distinction in the purpose. I would say. So the purpose for science is to build new knowledge that we have and then you can share the knowledge in papers or in prototypes or in other ways. But the purpose for engineering is to provide value or build a product in a way. So then you know, to build a product or to provide user value in some way, you need to have knowledge, you need to have science. So you use science to be able to build the products and the value that you want to provide.
Speaker 2:But the purpose is very different and when you do science you need to have some engineering. You need to build like a prototype, you need to build up experiments. You need to build stuff to be able to come up with a new knowledge experiments. You need to build stuff to be able to come up with a new knowledge. But then you do what you need to do from an engineering point of view to you know, prove and build a new knowledge. Versus in engineering you use knowledge you need to to be able to provide the value from a product point of view, would you say that's a fair description.
Speaker 3:Yeah, I would agree.
Speaker 1:And then it's the classical conversation then difference between applied research or engineering-based research. If I take Google or Elon Musk and SpaceX, who are sort of a strong engineering culture, but of course they need to know how to research or they're figuring things out.
Speaker 3:No one has done before. So is that research? Someone would you know? Is that research or is it not research? I, I guess it's some some kind of applied research, but but, um, yeah, there are many types of research. You can do pure theory, or you can do pure studies, where you have to collect data and look at the statistics.
Speaker 1:It's a spectrum, right, yeah, but it was this funny beef between Jan LeCun and Elon Musk. I don't know if you follow this, but this was such a. I think Jan LeCun went a little like snobby in terms of it's not research if you're not published, you know, and Aaron more or less said get fucked, we're doing cool stuff.
Speaker 3:Yeah, I saw some discussions about this. They have some. I think they call it Neuralink or something that they claim that they can put electrodes or wires in your brain they actually have that done, done that in a human now. So yeah, but but I mean, I mean that's a whole research field of its own, and and to claim that you are better than all researchers in the world on that topic, it's a bit arrogant and that might be true, but but are they doing research on your link?
Speaker 1:doing research, what are you doing? Because they Are Neuralink, doing research Because they have clearly a product. This is the point. Right, they have an engineering product idea, they want to build something, right, but they are doing something on such a bleeding edge. So they are sort of competing with the best research institutes on understanding how to do this. And I think this is very interesting, especially in the field of AI. Who is the beating edge on research in AI?
Speaker 3:I mean, yeah, I suppose they are doing research in one way, but but if, if they never publish it as you say exactly, then no one else can know exactly what they did or they cannot continue the work. So, from that perspective, it's not research, I think you.
Speaker 1:I think you put, I think you nailed it with that comment right, Because this is how I can understand it also One is in the pursuit of knowledge and one is in pursuit of product superiority, so to say. And hence, of course, you're both doing research, but one is science one is not. Maybe that's the way.
Speaker 2:Yeah, but I think you can still do science without publishing it, can you? But can you call it science? Maybe that's the way? Yeah, but I think you can still do science without publishing it publicly?
Speaker 1:Can you, but can you call it science? I think that's a fair question.
Speaker 2:I think you can have it inside an organization like a pure industrial tech company. They can conduct research, they publish it perhaps only to themselves not to the research community. It's still building knowledge and the people doing that internally, like some companies starting with A, then they are, I would say, working with science, but they are not sharing it, and it's still like the purpose is still to build knowledge, but it's not shared.
Speaker 1:Yeah, but we get into a semantic war here, definitions and all that. But I think it's quite interesting.
Speaker 3:Yeah, but we get into a semantic war here. You know definitions and all that, but I think it's quite interesting it is. I attended this big NeurIPS conference last year. Oh you went there Nice yeah, and then they had this sort of discussion where I think persons from Openea were part of the panel, but in several locations locations they said we cannot say how we did it. And then some in the audience started to complain yeah, but this is an academic conference, we cannot say what we did.
Speaker 2:That's a good question. Is OpenAI, which is not very open, actually conducting science or engineering? And if they are not open with it, they're at least not doing academic research. I think we can all agree on that.
Speaker 1:I think there's clearly, then we need to start doing definitions in a spectrum that we have the hardcore, the pursuit of science is the pursuit of knowledge has to be shared, otherwise I could not agree with that. This is sort of science it is not to me.
Speaker 1:And then you have the full other spectrum where you have sort of product development, super proprietary, and then we have a gray scale in between, right but but there is, there is there's a standpoint to me that goes all the way to this spectrum and all the way to that spectrum. I'm not saying we shouldn't have this stuff in the, in the gray zone, but I kind of think you need to be more nuanced on how you discuss this, then I think also the same question about you know sharing or not can be applied to engineering yeah, so you can have engineering that is not shared at all.
Speaker 2:It's still engineering. But then you have the full open source movement, which is also engineering, but it's heavily shared. I think both are engineering. I would do the same kind of argument for science. You can conduct science that is shared or not shared, and you can conduct engineering that is shared or not shared. It's just another dimension.
Speaker 1:Okay, so then we have another definition of open versus closed research or open versus closed science.
Speaker 3:Yeah, I mean, I think that the research field in general is going more and more to open science, because there are, but is it really? Well, it's not very fast, but I would say slowly because, for example, the funding agencies are demanding more that you publish open access and that you will share your data. This is slowly.
Speaker 2:But still, this is a very interesting topic.
Speaker 1:We've been going down a rabbit hole now, so I'm fine with that by the way.
Speaker 2:But if you then argue that more and more of the big tech companies are driving research or science, perhaps universities and academia is not to the same extent, at least anymore. If you go to NeurIPS, it's easy to calculate the number of papers coming from universities versus companies, and I think we can see a very strong trend moving more towards tech companies. And then you can question okay, Google at least is very good at publishing and being open, so I think they are at least trying to be open. Some other companies I won't name the negative one, I've probably said this many times already, so you know what I think there- this is an off-work topic.
Speaker 1:We will take with. Anders, we're going to make a list together with us.
Speaker 2:In short, I wish we could have more academic research. I feel really bad about that. The academic research is not having the power that it once had.
Speaker 2:And if you take funding, there is no, no comparison. I would even say if you take funding coming from, you know tech companies and how they can fund their own research compared to universities, especially in the field of ai and the llms and the large foundational models, no university can, you know, build foundational models anymore right now, it's extremely hard for a single researcher to compete with open ai or yeah, yeah, but there are, I think, more and more open language models like this mistral, or what it's called yeah and so, and in sweden we have this berserlius cluster that anyone can use.
Speaker 3:It's like a thousand gpus and then you have xai.
Speaker 2:They just released a hundred thousand h100. It's a, it's a mag, three orders of magnitude larger. Yeah, the same with meta, microsoft and google. They all have like a thousand times per series.
Speaker 3:Yeah, playing with no I I don't think we can compete in terms of uh hardware or but we can make. No, no, but we can maybe compete with uh or money. No, but we can maybe compete with using smarter methods or smarter models, or potentially, and I think also potentially more unbiased research questions.
Speaker 2:So it's not driven by monetary gains to the same extent as the companies are. So I think it's super important for universities to be successful and I wish we could have more efforts helping academia to be more powerful, but I think the trend is going in the opposite direction, unfortunately.
Speaker 3:Well, I think that this WASP program, that they are donating a lot of money.
Speaker 1:WASP, I think is something unique, so that really helps Sweden yeah.
Speaker 3:But it's also a bit sad that the private family has to do it.
Speaker 1:Yeah, it's an interesting statistic when you look at the funding from WASP compared to on the other side.
Speaker 2:We're having an oil fund in Norway driving so much research as well.
Speaker 1:It's also kind of strange.
Speaker 2:I wish we could have the governments really stepping up here and helping academia.
Speaker 3:I think they have decided to increase the funding for research the coming years. But at the same time they haven't adjusted for inflation in like 10-15 years.
Speaker 1:So we're going back to normal. Should we go back? I was thinking a little bit. If we're spinning on this research topic and now you're going into this call that you applied for with WASP, what is the research angle or what is the things that you really want to explore?
Speaker 2:what are the research questions in the?
Speaker 3:application exactly.
Speaker 1:It's similar to what we research angle, or what is the things that you really want to explore what's the main research questions in the application.
Speaker 2:In the application Exactly.
Speaker 3:Well, it's similar to what we discussed, but one question is how do you merge the models in the best way? You can call that aggregation function. So what are the best aggregation function for different models, for different heterogeneous data?
Speaker 1:Exactly what we talked about before. So this is now the aggregation function pattern we are talking about Fantastic.
Speaker 3:So that's one aim. Another aim is how can we harmonize the data or, for example, it can be images or genetics or text. How can we harmonize the data so it's more similar?
Speaker 2:So normalize them in some way or regularize them in some way, or what do you mean with harmonize?
Speaker 3:Well, maybe one city has an older MR scanner, so maybe the images are lower quality or lower resolution.
Speaker 2:And then perhaps different age groups, as you said, or different demographics or different hardware devices.
Speaker 3:Yeah, and if you work with text data, maybe there are different languages, bit of different languages, so then maybe, maybe one word will not mean exactly the same thing, even if you translate it.
Speaker 1:it can be worse than that different hospitals have slightly different ontologies or definitions of things in their journals yeah, yeah, I mean like this is not perfect.
Speaker 3:Yeah and in. In radiotherapy there are different clinical guidelines in different hospitals.
Speaker 1:This is my point. This gives different ontologies, for sure.
Speaker 3:Yeah, so to make the federation work better, you need to harmonize the data to make it as similar as possible, but then the challenge is to do that without sending any data around Tough so that sort of.
Speaker 1:I've just been trying to wrap my head around the fundamental topic of bias and what happens with bias as a problem in federated scenarios. Could we elaborate a little bit on the fundamental topic of bias? We've heard about it, we've talked about it. How do we need to think about that in a federated context?
Speaker 3:Good question.
Speaker 2:And just to elaborate a bit more, I mean biases. In itself is an essential.
Speaker 1:It's a feature, not a bug.
Speaker 2:I think we need to differentiate between good and bad biases, thank you. So some biases are good. It helps us tell if it's a bad or malignant or benign tumor, for example. Other biases, perhaps based on age or whatnot, or race or whatever, perhaps should not be used to try to do these kind of predictions.
Speaker 1:But if age is a biased topic, that is relevant to understand this is a perfect example right. That we want to understand that blacks are getting more cancer, if that's the reality.
Speaker 2:That's very true. Bad example for me, yeah so bias is difficult, it is so if you say that we want to harmonize the data and I guess from a hardware device quality kind of point of view, it's easier to understand we want to have you know the data looking similar, independently of the mri scanner that you have or whatnot, but when it comes to demographics perhaps it's harder, right.
Speaker 3:Yeah, I guess it's not really possible to change the age of patients and similar things.
Speaker 2:But I guess you could. Normally, if you just keep the data local to a hospital and say we want to average it out so it's always on age 30. And then if you have some global pattern saying this is the way you normalize it, so it looks like a 30-year-old, perhaps you could do it in some way. But you don't go that way. Way, you don't go that way.
Speaker 1:You wouldn't go that way. That's a stupid example, probably.
Speaker 2:But I guess you could try to. I mean, you don't have to share the data necessarily to potentially normalize it against certain dimensions.
Speaker 1:I have a super hard time wrapping my head around bias in relation to distributed approaches. What's the implication of that?
Speaker 2:I think it's.
Speaker 1:I don't understand. I haven't thought about that myself.
Speaker 3:Well, I mean how to evaluate federated models. That's sort of a story of its own.
Speaker 1:Yeah.
Speaker 3:But you, I mean you can either have a validation data set in every city or you can have a separate city. That was not part of the training at all. So how to evaluate the global model is not obvious. So you can do it in so many ways.
Speaker 2:But I mean, do you think it would be okay to, for example, just share the distribution of different parameters like demographics or the hardware device used, and then, if you send that and share that, perhaps that's still keeping privacy safe to some extent?
Speaker 3:Yeah, I suppose that you could. Instead of only using the image data, you can maybe fuse it with demographics like age and sex.
Speaker 1:But one way of looking at the same problem is like is there a fundamental difference in understanding and working and managing bias in a more monolithic solution versus a distributed solution, or is it the fundamental same problems, or is new problems arising? This is maybe the core question.
Speaker 3:I have not thought so much about it, but there's an interesting yeah.
Speaker 1:yeah, this is one of those things where people are hard. Someone would argue around these topics without having a clue, right On top level. Right, Maybe it's the same fundamental process.
Speaker 3:I think it's the same fundamental problem, but it may be harder to measure it.
Speaker 1:That's the point.
Speaker 3:Because there are so many other things that differ.
Speaker 1:That creates noise.
Speaker 3:Yeah, that would be my first guess.
Speaker 2:Interesting. So to just summarize the topic a bit here and trying to understand what the main research questions are in the application, partly is we need to merge the application or the model somehow and do these kind of aggregate functions in as efficient way as possible. You also need to harmonize the data, as you say, in different ways to make it independent of what the potential bad biases are in each site that you have, or silo as you call it. Is that the main one?
Speaker 3:silo, as I call it, um, is that the main one? Or is that the main like? Uh?
Speaker 1:well then, we have um one aim, about um data privacy and data leakage so yeah, do we want to quantify data leakage, memorization, so you want to continue on that what someone else said. You, you mentioned a paper, you mentioned there is a topic someone had to try. Try to look at leakage in a different context the Halland-Cossenberg case.
Speaker 3:Yeah, there are more and more papers on, for example, diffusion models that show that, for example, diffusion models that can create synthetic images, and we found the same problem ourselves that instead of generating new images, it will just generate images from the training set, and that's not really what you want could you elaborate?
Speaker 3:I didn't follow that yeah, if you a diffusion model is kind of generative model that can learn to generate, for example, new images. So if you train it with, say, 10,000 real images, it can learn to generate new images that look similar or come from the same distribution of images. But several papers have shown that it's sometimes likely that it will not generate new images. It will just take one of the training images and make a copy and then you have not reached the goal of generating new images. Then you're just making copies of your sensitive data.
Speaker 1:And do we need to make a small comment on the difference between transformer models and diffusion models here? Because as a layman I understand. With diffusion models it's quite different techniques. You're introducing noise and then you're going backwards.
Speaker 2:Yeah, diffusion models can use transformers as well.
Speaker 3:Yeah, transformer is more about the architecture backwards, or yeah, diffusion models can use transformers as well. Yeah, yeah, transformer is more about the architecture okay so you can have a diffusion model using what we call convolutional layers, where you do something called convolution, or you can do diffusion models with transformer architecture okay, so it's.
Speaker 1:So. I'm mixing it up here as a layman.
Speaker 2:Please help me, please save me, I'm going to do some things, but but I remember speaking to scale out and andreas there for a while and I don't remember exactly the wording he used, but in terms of keeping the data safe and data privacy, as you mentioned. You can think of techniques going into the model.
Speaker 2:You can do stuff like differential privacy, for example, like trying to add noise to the data, to remove potential PII or personally identifiable information to it, and homomorphic encryption and whatnot. So there are different ways to do it when getting to the model, in, so to speak, the inflow of data to the model. But then you can also think potentially data privacy model out. So that means basically that the predictions you're making from the model could potentially leak the data you have. You're making from the model could potentially leak the data you have. I remember some kind of article trying to see if they can, from GPT-4, see some kind of text paragraph that was included or not, and they could actually extract to a rather high degree these exact quotes that's been used in training for it, so they can extract from the model the data it has received, and that's both bad from a privacy point of view. Yeah, is, is this? Um, I guess this is some kind of research questions that could be interesting to look into as well.
Speaker 3:Right, yeah, I mean we. We propose that we want to take our trained networks and see if we can reconstruct the images that were used to train it.
Speaker 1:So you're really hardcore, trying to see if you can get the leakage proven.
Speaker 3:hardcore image yeah, and so that you can quantify how close is that to a real image from a patient. So you can get some hard numbers on.
Speaker 1:That's a valid question. It's a good question.
Speaker 2:So is that vertical model leakage in some way, or what do we call that?
Speaker 3:I'm not sure what the proper name is. You need to figure that out and you need to put a paper out at NeurIPS.
Speaker 1:Can we just move to the synthetic data kind of question a bit here? Proper name is you need to figure that out and you need to put a paper out at NeurIPS.
Speaker 2:Can we just move to the synthetic data kind of question a bit here, and let me, as a non-expert in medical field, just ask a perhaps stupid question. But synthetic data is used more and more, I would say, for also LLM training and foundational model training. Some people claim oh, we have no more data to train on. I think it's rubbish anyway. But anyway, you can see that you know, potentially it will come a point where we're starting to get a lack of data to train this kind of super large foundational models on. And now we have this kind of O1 model that is using potentially techniques even though we don't know it by heart because they're not very open in open AI. We can guess that they're using this kind of star or self-taught reasoning kind of approach, similar to AlphaZero.
Speaker 2:Alphazero was this technique back in the day in 2015-16, where they trained a model to play chess or go without using any human data at all. So all it was synthetic data. It basically played itself. So it generated its own data and tried to, by having some kind of game, see if it was good or bad. And some people now claim you know, this is actually what O1 is doing in some way. So they're generating data in that way, improving the model all the time. Do you think there will be similar kind of ways for let's just take semantic segmentation. Can you generate images to make the model improve itself without even using? You know real human data for it?
Speaker 3:Maybe. Well, we have published one paper where we show that you can train a segmentation network with only synthetic images, but without any human data at all. I'm not sure.
Speaker 2:Perhaps you have some kind of GAN approach, where you have some kind of detector and generator and it tries to fool itself in some way yeah, I mean we, we managed to show that we can.
Speaker 1:We can generate realistic images and segmentation itself at the same time, and then we can use that to train a segmentation network but have you read the article or paper on the I can't remember if they call it degradation or like what happens when we run out of human produced data and ultimately it's only training on synthetic data and ultimately there was a paper published in a couple of maybe a couple of months back or one month back where they were arguing that in the end, we will lose any quality.
Speaker 2:I mean it can be mode collapse. Yeah, it was a quite dystopian paper, but there are examples where they do the opposite, so they actually gain above human performance.
Speaker 1:With the synthetic data.
Speaker 2:I just remember one project we did in Peltorian time with Electa, actually. And we had this problem where the ground truth, the label data for segmentation in this case for actually. And we had this problem where the ground truth, the label data for segmentation in this case for actually, brain tumors were made by humans, but humans were inconsistent with themselves.
Speaker 1:So even expert radiologists. You know, they had only like 85% consistency or something.
Speaker 2:So we knew that the accuracy was bad because humans are bad. And then we had a model that could come up to 85% accuracy. But how can you even go above 85% when the training data itself has so much error in it.
Speaker 3:That's another aim of our project.
Speaker 1:Oh, really. Oh, that's a good one.
Speaker 3:Explain no, but that's a challenge in radiotherapy that since there are different clinical guidelines that say exactly how you should draw the tumor, the data will be different from different cities. So then we have proposed two ways that can potentially solve that. That's super interesting. So one way is to include uncertainty in the model, so that you, for example, say that you should be very certain at the center of every segmentation, but you should be uncertain at the border. Or, for example, that you can maybe, if you have a segmentation network with maybe five layers, you could maybe train the first two layers in the federation, but the deeper layers you maybe only train locally, or something oh, this is so interesting how you need to become more nuanced and you need to figure out how you do it.
Speaker 1:On the certain, this is everybody is doing the same, but the longer further out you get, this is more uncertainty and you need to have localized yeah well, we don't know if it will work, but that's what we but the research question is super interesting, yeah so it's, we have this it's time for AI News.
Speaker 4:Brought to you by AIHitW Podcast.
Speaker 2:We have this small break. I'm not sure if we told you, anders, but in the middle of the podcast we take a small break and just speak about some of the recent news that we had, and each one of us can bring up a favorite research article that we want to speak about or a news article in some way. Let me ask you, anders, do you have something that you would like to speak about, something you read about recently?
Speaker 3:Yeah, I recently read about a news article and it's actually about medical data.
Speaker 2:About what Medical data?
Speaker 3:Yeah, so it's about transcribing About what? Medical? Yeah, so it's about transcribing conversations between doctors and patients, and that I mean. I've never worked as a doctor myself, so I cannot say how much time it takes, but I guess it's something they have to spend a lot of time on, on writing down the main points of this conversation. So there are apparently now software you can buy that will do this automatically.
Speaker 2:The Whisper model from.
Speaker 3:OpenAI is amazing.
Speaker 2:It's prescribing audio to text.
Speaker 3:But the problem that was mentioned here is that Whisper will sometimes hallucinate, so it can add parts that were never said in the conversation okay, so the article was about that, yeah, yeah, so it can, for example, add that someone was violent, or it could add a racist, racist comment or Hallucinating full on yeah.
Speaker 3:Wow, so then it got me. I got very confused, because normally if you want to use software in a clinical setting, you have to go through many steps of validation to get it approved. Yeah, and according to this news article, it sounded like it should not be possible to get it approved. If it hallucinates, so either they have not done a proper validation or they're using a software not clinically approved, which is also strange.
Speaker 2:That sounds super strange. It's a very interesting topic, I think. I guess also the it sounds strange. I was trying to find an angle against what you said, but I can't really do it. It's very true what you said. How can you not? At least, yeah, I don't think.
Speaker 3:and it also said that when it has transcribed the audio, it will delete the audio. Oh so you cannot even check. That's even worse, um. But but then I read that this nabla company commented that we we haven't seen a lot of hallucination. So then I'm not sure what to believe but.
Speaker 1:But it sort of puts the finger on that we have so much opportunity to do things that augments our workflows but we kind of need to do AI innovation, ai safety by design. At the same time, I came to that conclusion in other angles that AI innovation, ai safety kind of you need to do it by design, you need to think the right way from the beginning, and AI safety kind of you need to do it by design, you need to think the right way from the beginning.
Speaker 3:Otherwise there is so much blast radius in all this stuff. There are several persons that complain that the United States, they innovate and the European Union regulate. But here it seems that we should have more regulation if they use this hallucinating model in hospitals.
Speaker 1:I think the fundamental argument is can we find a path where AI safety and AI innovation converge? So the whole sort of bipolar understanding of this is wrong. So what is the middle ground? That is the right way, but for me, the bipolarism in this argument is wrong. Both extremes are bad.
Speaker 3:Yeah, you need to think of it from the start.
Speaker 1:So for me this becomes a by design. How do I design my process? How do I design my research? How do I design my product development as a way, so I balance or iterate between these perspectives, because if you do innovation as an afterthought, you're fucked, and if you do regulation as an afterthought, you're also fucked, in my opinion. It's that simple, sorry for my language, but it's so obvious. But no one gets it right in my opinion. But it's a hard problem. No, I don't think it's hard, it's by design, balancing.
Speaker 1:No one has figured it out in a good way, yeah, but iterating between two extremes is a two-hat problem. You need to wear two hats. You need to flip hats iteratively.
Speaker 2:I don't think it's that hard. I think it's more political. If no one has found a solution for it, then it's probably kind of hard. No, it's probably kind of hard.
Speaker 1:No, it's okay Theoretically not hard. Politically, organizational people practically hard. Engineering-wise probably hard Engineering-wise yeah. So practically hard In theory. If you have the right steering on this, I don't think it's that hard. I think it's hard, but it's an important one. It's an important one. Do you have one, henrik? I have an important one. It's an important one. Do you have one, henrik? I have an angle now.
Speaker 1:I have three different articles that I find I just found a new cool guy, ryan Dawes, is one of those tech editors or tech writers and he's doing a pretty good job of doing nice snippets of AI news. So shout out to Ryan Dawes. And I find the one I want to lead on is, I think, a very interesting one used for people to know about. So the Open Source Initiative, osi, has unveiled a definition framework to evaluate whether AI systems can be classified as open source. So the announcement of the first open source AI definition O-S-A-I-A-D OSAID. So it's the whole thing about.
Speaker 1:We have had the conversation about oh, we need to go open source on models, but what is open source and who is open source? It's a minefield, right. What is really open source? And I found that very refreshing that someone is trying now within the OSI framework has been around quite a long time, I think to support these topics. You know, to understand open source community. So they have a definition that I find is quite interesting. So an open source AI system must be made available under terms that grant four essential freedoms Use the system for any purpose and without having to ask permission. Study how the system works and inspect its components. Modify the system for any purpose, including change its output, and share the system for others to use, with or without modifications, for any purpose. So it's an interesting, it's a quite concrete. You know, trying to take the open source ideas from software and how would we apply it to ai? And if you follow that definition, as that is truly open source, I don't think it's that many.
Speaker 2:Very few.
Speaker 1:Very few of the models would actually fall in would be labeled open source. So we get to the point. Once again. It's a spectrum, right, but I just found that to you know.
Speaker 2:But it's interesting how it works. It's even a part of GDPR. You have to be transparent how the model works. If you want to use an AI model in that and it's kind of funny how do you do that for a deep learning model you can say that here are the parameters. It's just a mathematical procedure. Here is how it works. Is that sufficient? Who?
Speaker 3:knows.
Speaker 1:But my point with bringing up that particular piece of news is that this is a big topic, I think, in terms of understanding that, and I think even in this room we have swayed back and forth, Are we? You know, large language mods, all that thing, we should really try to make it open source. And then we realized, oh my God, how powerful they are. Can they open?
Speaker 2:source. I think you know, with this definition, fewer and fewer of the foundation models are getting open source, you know.
Speaker 1:I'm not even sure the meta ones would fall into that category. I don't think so Interesting, right? So that was one. I had a couple of other ones. I can do one more if you have time. The other one, this one I thought was fine. Same guy.
Speaker 1:Mit Breakthrough could transform robot training. So in MIT researchers this was sort of the news. I don't know when the paper was out, but the news flash is from the 29th. It's new. So they have figured out a way how we can improve and develop robot training methods that reduces the time and costs while improving adaptability.
Speaker 1:So the core problem when you're training robots and when you're training is that it's always done very specifically. So they are trying to find a generic approach where you can get data from any kind and in this way then structure it so you can use it for training robots. So the approach is called heterogeneous pre-trained transformers HPT, and it combines a vast amount of diverse data from multiple sources into a unified system, effectively creating a shared language that generate AI models that generative AI models can process. So it's some way in sort of using generative AI, using the large language models techniques, as a way to sort of simplify finding the specific training data set for a specific robot application. I just found it interesting. So I mean, like, so, if you think about what are the different research angles, how do we truly progress so you know how to get the cognitive AI with the physical, so to speak? And then this, I guess, is a big hurdle, how you train the physical robots. And now they think they have made a breakthrough on how to think about that. I found that interesting.
Speaker 3:Yeah, I've read that Jan Lekund keeps using this example that LLMs cannot reason that it cannot understand basic physics. I don't know if that is correct, but he keeps using this as a counterexample of that LLMs can reason Because if they would understand physics, they would know what happened if you push an object.
Speaker 2:Yeah, and I think what he's saying. I follow Jan Likun so much. But at least if they only have textual data, how much can you really infer in terms of gravity?
Speaker 2:or physical laws that we otherwise have. You can get some part from text but unless you have visual data or multimodal data, some parts will not be captured. But I think you know some other people have said that even the concept of colors, which seems hard to get by simply looking at text, seems to be captured still by, you know, purely llms that only see text, that seem to have some understanding of what colors are, which is kind of interesting. But of course you can. Some concepts will be super hard to capture without having, uh, image data to com to combine with textual data.
Speaker 1:I think yeah, we can stop there, but there were two. You know two, two interesting pieces.
Speaker 4:I I have a few um, because I think that we have missed some of the most important happened last week, so I'm gonna be just very quick about it. First of all, denmark became like a superpower yeah, this is good, because they they got their own, jeff Jeff.
Speaker 2:Jeff Jeff, jeff, jeffion, jeffion.
Speaker 4:Jeffion super computers and Jensen was there and they promoted actually the launch of the AI supercomputer in Denmark together with Prince or King the Danish King was joking.
Speaker 1:I guess there's two Kings in the room. That's very true. I found that a refreshing comment by a monarcha, by a mona. I guess it's so cool, it's very cool.
Speaker 4:Um, as you know, my news always about usability and stocks and everything else. So, uh, the first thing, it was the negative one. You remember there was this accident last week where one kid in the us, I believe, committed suicide by just basically talking with the chatbot from a company called Character Technology. So they have, like this AI chatbot that you can actually talk with. It's very popular amongst children and it's basically giving them comfort and etc. So it came to that point that the chat was basically very, very emotional and providing some sexualized conversation and encouraged suicidal thoughts and then the kid basically killed himself Terrible.
Speaker 4:It's terrible. So now the mother is basically suing them and probably she will win, because now, when they're looking at the chatbot and how it's built and et cetera, it's actually very addictive, as they say, and it exploits children, leading to an emotional abusive relationship in a sense. Right. Third one you remember the linkedin? There was a big bond like that. That linkedin basically just enabled the collections of your data without even noticing you and casey, yeah it was a massive outlaw yeah right from the people who understood it yeah, good thing is that we in Europe, we were protected from that.
Speaker 1:That was only in America, yeah exactly America.
Speaker 4:So now it came basically why they were doing it. So they are right now releasing AI agent, which is basically going to help recruiters to select candidates, candidates and then also help them target the LinkedIn users as effective as possible, matching them with their you know criterias and everything else. So now they are making a proper business out of it, and LinkedIn, for a long time, has been actually making a lot of money from the recruitment side. It has been their strong side in the capitalization of the entire product, which I think is super good. Yeah, then I will leave this for the next time, which is basically IBM Granite 3.0. Right, because I will leave this to Anders to explain this for the next one.
Speaker 4:And the last one that I think is very interesting is this one is basically a cost of sleeping on AI, which many people are still not believing that this is not it and it's not a big thing. This is what happens with companies that are super big and they are basically late on the AI journey. So Samsung loses one third of its value in four months. Basically, $122 billion of the market value was erased since its peaks in July 9. And this is actually expected because they were very late, july 9, and this is actually expected because they were very late.
Speaker 4:We are talking about the semiconductor part of Samsung and not basically the, uh, the phones and etc. Right, so, um, it's uh, sad to say so. This is basically explain everything. We know that the Nvidia is on the top of the game when it comes to chips and, uh. We know that Apple and TMC are gaining quite a lot of traction in that, and TMC alone has added over $330 billion in the market value this year. Well, yeah, samsung has been lagging behind it and you see what has happened One third of the entire value deleted just in a matter of months.
Speaker 2:It is a very interesting moment and you can see. Basically, of course, csmc is a leader in this, with 90 plus percent of the advanced chip market, but you can also see all the tech giants now producing their own chips and Samsung is not doing it. So it's possible to compete with NVIDIA and TSMC, but Samsung has failed.
Speaker 4:Yes, has failed. It actually has not been on time Sleeping on it. Yes, sleeping on it costs, and that is basically now we know what is the KPIs of sleeping on it. Yes, sleeping on it costs and that is, uh, basically now we know what is the kpis of sleeping on ai. Can you, can you?
Speaker 1:extrapolate on that sleeping on it from the chip market and this context can you extrapolate all the way out to using ai, you know, in enterprise and all that? Can this, can this sort of be? Can we see this as okay? This is sort of giving a trend, a signal, a trajectory that if you are, this is what happening further back or upstream. So if you go downstream and we are now becoming software companies or becoming enterprises and we are sleeping on it, will we have the same sleeping on the ai effect all the way down the downstream?
Speaker 4:I think. So I will let you discuss this, but I think there is like a two. It's a palette of how much it's going to affect you. Yeah, true it's great because if you look Samsung, their core business is semiconductors, which means losing on this is one third of it, but for example, for Scania, or Ericsson it's a little bit indirect, because it's hidden inside of the optimization of their processes and customer service maybe.
Speaker 1:But my argument is because it's indirect, it's a lagging effect, but the effect is the same.
Speaker 2:Yes, exactly the statement saying you know, the AI won't replace humans or companies, but ai or companies that use and people that use ai will replace companies and people that don't use ai, and that's certainly true for samsung and I think it's true for most companies. It's just a matter of time before it happens in some way.
Speaker 3:Well, jeffrey Hinton claimed that I think seven or eight years ago, that medical doctors who look at images would be replaced by AI, and it has not.
Speaker 2:I think that's the wrong way to see it. I think it empowers people and radiologists and other people that use. Ai will be the one that.
Speaker 4:They can work more efficiently, but they I don't think we should replace them look at the doctors today they're spending maybe one third of their time basically just uh, um, going through the journals or what they have done today and they need to basically record that and that goes to a repository system that somebody's typing that and everything else right, keep so if they basically utilize this time to actually save lives rather than actually doing, you know memorizing what has happened in every, each second of their daily work.
Speaker 4:Only that is actually beneficial, right? So in that case there are more. People will have opportunity to focus more on what is their core business rather than actually doing the admin stuff and everything around it.
Speaker 2:You've already taken so much. I had a big topic that I want to bring up, so now I have to really compress it into a few minutes here, which I think will be really hard. Go for it, okay. So one big release in last week was Claudeai Anthropic. They released a new version of the 3.5 Sonnet, and the Haiku Sonnet is the mid-size. Haiku is a small-size model that they have, so the new version basically beats GPT-4.0 on especially coding tasks to a large extent. So it's surprisingly good. But that's not the big news. The big news, I would say, is that Claude and Atropic have released what they call Computer Use.
Speaker 1:Yes, that's one. I saw that one.
Speaker 2:And I think this is more profound than people think. This is almost like a Terminator Skynet kind of moment.
Speaker 1:This is the agent story. It is Now we get this happening now it's a meta agent story.
Speaker 2:Yeah, for sure.
Speaker 2:So in short, what they have done is they trained the foundation model to not only produce text or images but actually to take action on the keyboard and mouse so it can control your computer, the keyboard and mouse Exactly, and it seems to do rather well. So what you do is you basically prompt it and say I want to give it a number of examples. They gave it a sample like I want to have some kind of sightseeing examples in the weekend, when my friends comes to san diego, and then, you know, you leave over the computer to the and it starts to open up the web browser. It can open the browser itself. It types in Google search saying sightseeing events in San Diego.
Speaker 2:It reads the result, it takes screenshots all the time so it sees what's happening on the screen and it can control the keyboard and mouse. And then it gets some output. It's summarizing them and finds these are the top events, and then it open up the outlook calendar or google calendar and it enters in a new item in the in the weekend. Here is the sightseeing events you should do, and it can then, you know, start using the applications and the interface that you must otherwise use on the computer and do that in a much more general way. And then there are much more examples like creating code and you can ask it to create a web page. Open VS Code, the coding environment, and it starts to run stuff there and it can see when errors happen. It can fix the error and then start doing it.
Speaker 1:This is mind-blowing when you start combining what, what this means yeah, and then of course, this is the first version.
Speaker 2:Actually, apple did something similar. You know apple has always been late on ai and machine learning, but they have apple intelligence, especially that version of machine learning. But they actually had something called um what was it?
Speaker 2:screen awareness or something so they actually watch what you do on the screen. And this is actually related to your field as well, anders, which is they want to be privacy conserving, so the data from the mobile phone don't leave and goes into the Apple Cloud. So by just watching the phone and what's happening on the screen, they can recommend stuff by understanding what's happening. So this is something similar, but I think in a much more general sense and much more profound. It's basically saying that if you want to take actions, instead of training a single action of I'd want to do a web search or I want to use a calculator to do some kind of math operation, it now goes a step back and say I can do meta operations, I can do whatever application I want and now in in the next sense.
Speaker 1:Now, now you can create your agents where you, where you define different agents or you have a meta model, you have an sdk for this and within this, and you can then and it can even go to other lms.
Speaker 2:I saw some example that said you know it actually opened up chat gpt so the lms opening chat gpt to get an answer to how should you do that? And they use the answer from the lm to take further actions. This is actually very profound, I would say it sounds cool, but very scary also yeah, but it but it shows the trajectory of what we are researching.
Speaker 1:When we say agi, sometimes it feels like it's somewhere out there, but what we're talking about is moving up the abstraction layer of problems we are solving and the sequencing steps we are taking, and the fundamental topic of agency. We're moving away from only prompting to actually actuating things, doing things, acting right. And agency here is the major topic.
Speaker 3:I think I would like a language model trained on my emails that can reply to all my emails as if I did it, so I can do research instead.
Speaker 1:Exactly, that's a fantastic bot and even take actions to research.
Speaker 2:Research.
Speaker 1:To answer the email properly you are not so far away from an avatar thinking here. This is, this is avatar land very soon, and even though this is first version. It works surprisingly well that's the crazy stuff, isn't it?
Speaker 2:and if you, I hate to be a doomer here but I have to give like a doomer example of this this is actually very similar to the Terminator movie.
Speaker 2:In the Terminator movie you had Skynets, some kind of defense system against nuclear attacks, and the problem with the defense system was that humans were too slow to see when actually someone is launching an attack. It takes too long time and it's too much data for humans to actually look into. So they said, okay, let's now give, let's take the humans out of the equation, let's give the full power to the Skynet computer to much quicker react to something happening.
Speaker 2:You're a Skynet nerd, you know the plot. You basically push the button and you push a button and that time you know humans are taken out of the control.
Speaker 1:But James Cameron, he was sort of envisioning that this agency this is exactly his argument.
Speaker 2:Now we just prompt the Claude, you know computer use feature. But if you were to just remove the human and let it prompt itself?
Speaker 4:it's actually a skynet moment in some way, we heard that there was an article that most of the geniuses in the world they actually tend to look at the history and the literature and sf and etc. And then they romanticize this and so when they're creating products, they're actually creating products that were their imaginary.
Speaker 1:But yes, this is the whole sci-fi romance and the tech bro combination the tech bro. So they will have this romanticized sci-fi topic. I've seen articles like this on Elon Musk and there are many examples of that that their utopia is not a real utopia, it's their. It's their adventures reading as 12, 12 year olds into Asimov or into. I've seen that comment.
Speaker 4:Yeah.
Speaker 2:Yeah, tech bros.
Speaker 1:Yeah, cool stuff, I think that's also scary, but but okay so that's also scary, but okay so. But I, I I went on the ramp page. I you're not. I've been on a rant or a journey where I think agency and agentic ai matters, and we we even talked about it in the opening season and I was actually.
Speaker 1:We had luca here, you know, and and I happened to hear a conversation or a keynote from him where he talked agentic, agentic, agentic in the spring, and I've been doing a lot of research on this in terms of what does that mean in terms of enterprises organized. When we are elevating the machine to coworkers, we get a principal agent relation with our machine. You know we are not organized for that, I can tell you that. So I think, pat myself on the back, I'm on the money here. This is profound stuff that will have huge impact in how we organize work, how we organize business ultimately. That's why to follow agentic, what do we mean with agency? What do we mean with agentic workflows? What are we talking about here? What are we actually doing? Super important to understand where it leads to.
Speaker 3:Yeah, I attended a small it was a small BOSP DDLS conference in Gothenburg and it was one presentation where one researcher had developed some kind of automatic researcher along the same lines.
Speaker 1:Like an avatar.
Speaker 3:Yeah, it proposed what should be the next experiments to do, and then it did experiments and collected the data.
Speaker 2:The AI scientist.
Speaker 3:Yeah, and then from that it draws some conclusion to do. And then it did experiments and collected the data. The ai scientist, yeah, and then from that it draws some conclusion to the okay, what are the next experiments? And so it could work itself.
Speaker 2:I mean, this is also, you know the levels that open ai produce. You know they had basically five levels and what they they started with was the conversational ai, the shapples that we have today. They're moving to reasoning. That's basically where we are today. We're starting to see it with O1. Next one is autonomous, which is basically agency. And then it comes innovating AI they call it and this is where they start to be more creative, perhaps more of the AI scientist kind of approach. And finally, the level five is what they call the organizational AI, saying that an AI is much more intelligent than a single human and reaching the scale of a single organization like Google or whatnot, in terms of capabilities.
Speaker 1:I think this is one of the better definition ladders of what we are understanding when we say general intelligence or general, because there's been different definitions on how do we define agi and there's another one floating around which has like a deep mind has one but I. But this one is very practical in terms of what the research problem is or what they are focusing on solving.
Speaker 2:So it's interesting as hell cool, scary but cool, I Okay. Getting back to the theme of the podcast as well, let's get perhaps a bit more future looking. What's going to happen in the coming years? What do you see are? I mean, privacy can be, of course, super important, and we're going to have to have ways of doing that, and federated learning is one part of that equation. But what would you say the main obstacles in coming years will be when it comes to, let's call it, privacy-preserving machine learning. I guess it's very related to the application you just made. Perhaps is basically what you just mentioned, but if you try to be a bit more speculative and say this is actually what I think is important to have privacy-preserving machine learning in the future, what would that be?
Speaker 3:You mean the most important application or?
Speaker 2:I mean if? If we think no, I think more like challenges in terms of being able to preserve date, human privacy or data privacy for humans while still having ai technology growing, as we have just spoken about in so many ways, but without having to have integrity kind of problems going forward. It can be anything from being inspired from music that is generated by humans that LLMs and foundational models can now easily produce themselves, or it can be more integrity-related questions or data security in terms of how can companies keep their trade secrets secret. What do you think the challenges will be going forward?
Speaker 3:Well, I think one challenge is I mean, let's say that you manage to train a good model using federated learning and that you want to make this into a clinical product. Then I've never done this process myself how to go from research to a clinical product but I mean you need to convince the FDA or the corresponding European organization that your model works as you want, and then they may say like, like, okay, but show us the data you used for training this model. And if that means that you need to bring all the data in one place, then you have lost the point of a dirty learning right if they required to see all
Speaker 1:the data you used you've lost the whole point. So you're arguing as well also that one of the biggest challenges and how will this progress, or how ubiquitous will federated machine learning become has a lot to do with how regulation is.
Speaker 3:Yeah, I think there's a big difference if you're talking about research or if you're talking about using it for real products.
Speaker 2:I think it's interesting. Also, I think you switched the whole question there, which is actually very good. This is a really interesting example. It's not, perhaps, how we can preserve privacy, but also how we can gain the possibilities that AI can provide, and for medical, clinical field. Clinical field that can be immense benefits of this, but that can be prohibited or challenged by the yeah, by privacy concerns, potentially. So, if you flip the question, how do we find the balance between still preserving privacy while still reaping the benefits, so to speak, of AI possibilities in the medical field?
Speaker 1:And being transparent and being explainable, and all this Because you have the balancing act. Now that we have XAI explainable AI coming in as a different force into this, that maybe pushes a completely different agenda.
Speaker 3:Yeah, I think that one key point is to be able to quantify leakage or memorization, because if you want to have discussions with legal experts or hospitals, they want some hard numbers on will it leak 5% or 50%, because otherwise you cannot even have a discussion. I mean, then they may just say no, just to be sure that it will not leak.
Speaker 1:They will just take the easy solution, which is to say no to everything but but maybe can I try another angle future Outlook, because I remember the first time I met Andreas, we talked about this and they started scale-up. This is about 2019-20. And to me, intuitively, I posed myself the question in 2020, and I want you to now answer what you think about this. Now I posed myself the question what is the future outlook for going distributed, federated versus monolithic? And my fundamental understanding from the energy ecosystem is that, damn it, the whole ecosystem is, by design, distributed. It's a network, it's an ecosystem, and here now we have techniques that are more centralized and we're trying to do that.
Speaker 1:So my understanding of this topic without going into any of the problems, any of the details is like use the trajectory. Are we moving from federated techniques or distributed techniques being a fringe activity to being much more normal? So what is the future outlook here? Are we gradually getting more and more into the distributor or will this continue to be sort of a fringe activity, because it's hard and all that? Because I had a view on this in 2020 and but I'm not so sure it's it's not so easy when I know more now. It's so many.
Speaker 3:I think it may depend a lot on each field, yeah, but I mean I don't see federated learning as the only solution. I see it as one of the solutions and then, like, synthetic images is another solution, yeah. So I think it will be one of the solutions you can use and it has certain benefits and certain drawbacks, and then synthetic images has certain benefits and drawbacks, so I think it will increase the usage. But I mean, I think there are more legal obstacles than technical obstacles.
Speaker 2:If you're facing a question like this, it has to be some balance in some way. If you have the quantifiable kind of obligation saying it's 1% or 5% leakage, if you require 0%, you know we have to shut everything down and we can never use any AI at all, would you say. Today, are we on the side of having too high requirements in terms of privacy or too low requirements to meet the benefits?
Speaker 3:If you consider what the Swedish authority on privacy, I think they are too strict Because you have to balance the risk to the benefits and I think for some reason I I think that sweden is in general too strict, like in the interpretation of gdpr, compared to other countries, and I don't know why that is why that happened. You know, maybe it's that Swedish people are more nervous or more afraid of doing something wrong.
Speaker 1:But it's interesting how you get so risk averse, so I mean like we had guests here who flipped the whole argument. How many lives should die? Because you know of this, is that ethical to allow lives? To get you know people killed because of privacy concerns. You know how do you balance this. You know what is the right balance here that we are. We are omitting to do the right thing.
Speaker 3:I think you should look more at the benefits yes and I mean one. What one simple way of avoiding GDPR is to only use data from people who have died, because the GDPR doesn't apply if you have passed away so if you you may still need ethics approval, but I think GDPR doesn't apply when you have passed away, so then we could potentially use their data maybe, but I guess a question could be what is the opportunity cost of not using AI?
Speaker 2:as you mentioned, and we have some guests on that before and we can see that if we use AI in this way whatever way that is then perhaps we can serve 50% more patients or something that could get helped and not have serious damages or even die. And if we at the same time then use AI, we may have some problems with privacy and perhaps some issues happening from that, and then you have to basically balance what is the cost of privacy versus cost of healthcare.
Speaker 3:I think recently they announced that I don't remember how many, but that people in Sweden had died because there were, or patients have died because there was not enough clinical staff to treat them.
Speaker 2:And if you can double the productivity we call it that of nurses and doctors that we have in the medical care, we could serve so many more patients, but we know we have a huge shortage in staff within 10 years.
Speaker 1:We know this from pension.
Speaker 3:We know we are getting into some really troublesome waters in Sweden, we have to use AI to save time in medicine. But then I think the key question is how much privacy are we willing to sacrifice?
Speaker 1:Because to me this also becomes not about. I don't like when you make this a bipolar or very simplistic question, because it's a nuanced question and and there can be many examples where you know you can, you can apply a framework where you you treat outliers in a certain way. You know there are many different ways to sort of do to balance this. So the whole black and white conversation I have so many examples there. I work at Vattenfall. Oh, we need to have the highest security because we have nuclear. Okay, so this application, now it needs to follow these security standards. And then I asked a simple question how many people are using that particular application? In relation to that, I need to put that security standard on my whole cloud. 26 people, okay. So you mean I'm going to put a technology security standard of 10,000 people because I have 26 outliers. Why don't I fix this in a much better way for the 26 persons?
Speaker 1:So what I'm trying to get to is the argument that why do we need to be bipolar? Why don't we need to make it black and white? Why can't we be nuanced? Why can't we find what is the real? You know? Okay, this is a security risk problem. Could you define that please? Could you go five levels down and then we can tackle each thing, and then I think we can get much further. But this is a much more sophisticated conversation, much more expert conversation, but it's not impossible in my opinion. It's simply that as long as we stay on this high fly level, we get nowhere. Do you agree with that rant?
Speaker 3:Because that's my rant. I think, as I mentioned, this interpretation by the EMI was too strict. They basically, as I understand it, they see any machine learning model as personal information.
Speaker 1:This is black and white right, it's a black and white argument.
Speaker 3:I think they are too strict, because that's the easy solution to say no to everything.
Speaker 2:That's of lawyer approach. You know you should even ask a lawyer if you're allowed to do something. Ask a lawyer. How can I make?
Speaker 3:this work or tell them afterwards no, no, no no, no, no, I I.
Speaker 1:I perfected this a couple of times in large enterprise, where it's how you preach the legal department, how you phrase the question. It's like because they cannot say yes, so you need to come with the case and you need to understand what do I need to fix? And if I fix all that, what do I need to fix now? And then in the end they run out of you know. So it's completely doable, but you cannot approach them in the wrong way, because then the whole thing breaks down in my opinion, but I think also you know we shouldn't be.
Speaker 2:I think we can have technical solutions to privacy and data safety concerns. I think the country and the company that works the most in this field will be very, very successful. So there are a lot of research questions that we should phrase and I think what you're doing on this year to improve that, to build more knowledge in a scientific way, to say that this is actually how we can have data safety while still achieving AI performance, which is what federated learning is doing to some extent I think that's super important and the more we can do work in this field to keep reaping the benefits of AI while still preserving privacy and data safety aspects, that's super valuable investments.
Speaker 1:And just to add to that, as you said, getting some hard numbers behind these theories, good or bad, means you can baseline, then you can start a real conversation. So sometimes the whole conversation lacks a baseline. So I think those kind of questions are super valid in a broader sense, in a broader sense.
Speaker 3:I can mention another project we are working on when we are exporting image data from 21 hospital regions. I mean, we have the same laws everywhere in Sweden, but so for every hospital region where we want to export data, you need like an approval and that sort of requires someone to sign off. But so the challenge here is that even if we have the same laws everywhere in sweden, each hospital region can interpret the laws in different ways. So you don't need to have one discussion. You need to discuss with 20 lawyers and they may have different opinions and interpretations.
Speaker 1:And maturity and competences, and all this.
Speaker 3:So, to have one discussion is one thing, but to have 20 discussions.
Speaker 1:This is how it works, but maybe this is also a part of the fix or the solution how this can become more efficient. Maybe this is where we need the AI agent right.
Speaker 3:It is right. I mean as or the Swedish government or the parliament needs to say this is. We decide this for every hospital, exactly so.
Speaker 1:It's the ambiguity and it's the lack of clarity that opens up, for I mean, like this is like when you do a strategy and you's the lack of clarity that opens up, for I mean, this is like when you do a strategy and you leave the tactical empty. You know you can build systems in 10 different ways. Everybody knows that. You can have endless arguments on governance or architecture.
Speaker 3:I think they I don't remember the exact name of the agency, but there was a governmental agency that contacted me one or two years ago and they wanted to do some kind of investigation on what is like the future of using, or how can we train deep models in medicine, seeing, or how can we train deep models in medicine, and if I remember correctly, their conclusion was the exact opposite of federated long, that they wanted a centralized server where you put all the data interesting and where everyone can log in instead and they saw they solved the topic of sharing data in a completely different way.
Speaker 2:Yeah, I'm biting my tongue Super interesting, I mean. We should be open-minded, though, in finding the best way to do whatever we can Do. You have any concerns with the AI Act, by the way? I think you know also what we mentioned before. For GDPR, for example, it's not really the regulation itself or the intention that is a problem. It's the uncertainty around it that causes companies and people to be afraid of it, and if we solve that, we can actually have a very good future with safety in mind, and to me, I'm a bit scared that the AI Act will have the same, you know, effects that GDPR, that it's the uncertainty of how to really comply with it that will cause more problem than the real attention. So what's your thinking about the AI Act?
Speaker 2:Yeah, my first guess is that it will hinder a lot of research and implementation, Because the medical field will be almost all of them in the high risk category right.
Speaker 1:Have you done that, the work you're doing? Now, if you look at your portfolio, how many of them are category nine or what categories will be your main categories that you will apply in? I cannot really say Because it's hard to judge, right? Yeah, it's really hard to judge.
Speaker 3:But in medicine there's another regulation called MDR for medical devices. That's also really tough to interpret.
Speaker 1:It's the interpretation and the opaqueness of all this that hinders people, and we had examples with GDPR. I think we have the classical Klaus Olsson case or someone else. I think we can mention them by now. It's Preskriberat, maybe not Fuck it.
Speaker 1:I did it. Someone basically said we're going to stop this because we don't even know what this is leading to. This is headache. Let's take away, we shut down our recommender engine because it's too much headache and this is what leads. I mean like if you don't have a big, it's a real consequence. It is real consequences that you know. You know what it's so many uncertainties. As it is, we might just spend our money somewhere else.
Speaker 2:I mean I hope someone do consider the opportunity costs. You know, in klaus wilson or some retailer you know, having, you know, not the recommender system in place and lacking on the win-win solution for both the customer and the company. Otherwise perhaps can be handled, but for the medical field we are speaking about human lives and I think this is something that we shouldn't really take or trifle with. I mean, it will have big impact if we don't do it properly and we need to consider the opportunity cost of this properly. Sad topic here no, it's an important topic.
Speaker 3:But it's solution-oriented. I heard like one week ago that it's a bit strange. But if you buy a clinically approved AI product to use in hospital, buy a clinically approved AI product to use in hospital, If you just use it out of the box, you don't have to apply for any permission or you can just use it. But if you want to make some small tests, like how well does it work on our patients, then you have to write a long application to Läkemedelsverket and explain what you will do.
Speaker 3:I mean so you actually want to do the right thing, which is to see how well does this work here in our hospital, but then you're punished that you have to write a long application instead of just using it out of the book, and just to make it clear.
Speaker 2:of course we all believe that regulation needs to be in place. We should have regulation especially in the medical field. So no one thinks otherwise, but it's important to have the right regulation and the right way to be compliant with it.
Speaker 1:I think no one is arguing for no regulation, and I think the important thing to get across as a match is the pet peeve that we've been pushing hard for several years is that we need to worry more about the how and the practicalities of this, and I even said that's a competitive advantage to Sweden. Klarna has this value proposition smooth payment so I was picturing smooth AI act positive thing, it is a competitive advantage. The one that actually gets.
Speaker 2:The one that gets this right. Yeah, that gets this right. As a country or as a company, they will stand out. They will have a huge advantage.
Speaker 1:So if you can create a self-service. We even had Petra Dalund here at RISE, so she's working with the test and evaluation facilityff, the teff around the ai act to set up a self-service for medium-sized business in sweden. I think it's a brilliant initiative and I'm like, okay, let's use poor money on that and make that into the beauty, most cool, coolest agentic app you can think of, right, yeah?
Speaker 2:That would be such a the ROI on that, you know.
Speaker 1:The ROI, the no brainer, the no regrets. So what should Vinnova invest in? The AI act agent, you know, in the TEF.
Speaker 2:With the sandbox or the testing environment.
Speaker 1:All this, all this, all this Something that helps companies to comply with this and making rice and you know, or a university make money out of this, instead of the lawyers and the consultants.
Speaker 2:Time is flying away. We are up to two hours already, so perhaps we should start rounding up a bit. And, anders, I would like to ask you a very concrete, no, a very philosophical question. I would like to ask you a very concrete, no, a very philosophical question. So imagine do you believe that AGI will happen Approximately?
Speaker 3:why? If so, I think it's very hard to say, but maybe in 10, 20 years.
Speaker 2:So, assuming that it has happened, and trying to think, you know, how will the world look like when it happens, then we can think of a spectrum. You can think about two extremes. One extreme is the terminators of the world where we have computer use and you know we will have a computer that is, considering humans to be a threat and they try to kill us all and you know, it's the matrix terminators kind of scenario.
Speaker 2:It's a horrible, dystopian kind of future, and the other extreme is perhaps the utopian one, where you think that we have AI that's solved cancer, for example, that you mentioned, or it has solved the climate change and the energy needs that we have and basically all the goods and services in the world are free of charge and we live in a world of abundance, as some people call it. Where do you think, potentially, we will end up? More towards the dystopian or more towards the utopian? What's your thinking?
Speaker 3:Well, I try to be optimistic, but yeah, I try to be optimistic, but yeah, I think it can really.
Speaker 2:I mean, I'm sort of a. Star Trek nerd, I'm thinking in the best case it can sort of help us to make starships and go to other planets, and they live without monetary value, right? They don't have salaries in that same sense as we do.
Speaker 1:What is the income approach? What is the in Star Trek?
Speaker 2:It's unclear, right? They don't have money in that way Does.
Speaker 1:Captain.
Speaker 3:Kirk make money or is he working for fun? They just do what they think is interesting, like doing research.
Speaker 1:They live in a Star Trek is a society of abundance. In that sense, right it is.
Speaker 3:I think, I mean, I think something like that would be the best case scenario, and I know that Jeffrey Hinton, he left Google just to talk about the dangers. So I think the main challenge is that we can go from stupid computers to very smart computers and maybe a few days like, like, if this automatic, what did you?
Speaker 3:call it reuse yeah, if that takes off, can take off like in one day or one hour, and then you cannot stop it if it's so fast that it but this is the fast takeoff scenario you're referring to. Now take my cast me, yeah, I mean that's the challenge that I guess it's really hard to know how fast will it happen exactly how fast will it contact other computers that also become self-aware and exactly, and perhaps you don't even need to be that intelligent to have this kind of fast take off in a bad way scenario happening.
Speaker 2:It can actually be a stupid ai yeah, that can spread very quickly like a virus. A virus is not intelligent, but it can be extremely damaging.
Speaker 1:We said, sometimes several times, we are more afraid of the narrow AI gone rogue than the general AI. That is actually.
Speaker 3:Maybe we should think like this Can an AI do more stupid things than Donald Trump?
Speaker 2:That's an awesome ending statement.
Speaker 1:You know what I'm all for the AI.
Speaker 2:With the election coming up, this will be a very apt question.
Speaker 1:That's a timely joke. I love it.
Speaker 2:Very cool. I hope you can stay on for the after-after work discussion when we turn off the cameras. But thank you so much, anders, for coming here. It's been a true pleasure to have you.
Speaker 1:So cool stuff you're working on, thank you.